Project Goal
To create tools for data analysis that aid in collecting plant imagery more quickly and efficiently.
Project Summary
Ian Stavness, Kevin Stanley, Mark Eramian, and their team of researchers are creating hardware and software for plant image analysis that will advance phenotyping and crop monitoring, as well as developing new methods for collecting those images. They are automating the types of phenotypes that researchers extract manually, and discovering new technological methods to generate phenotypes. This will help researchers automatically analyze images of plants and crops to identify traits related to growth, health, resilience, and yield. Currently, Project 3.2 is creating the software platform used to discover and measure digital phenotypes; this phenotypic recognition package is similar to those used for facial recognition in humans.
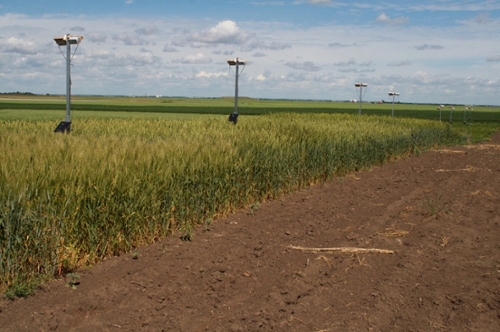
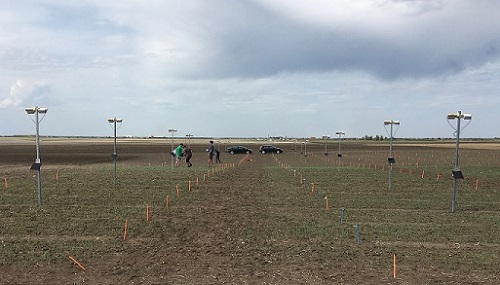
Project Results to Date
Project 3.2 has a few main focuses:
- Traditional image analysis involves preprocessing photos, segmenting the foreground (plant) from the background (soil), and feature extraction – assigning digital phenotypes or numbers to certain traits in order to better understand which traits correlate to important plant features.
- Machine learning is teaching a machine to automatically make predictions and utilize data in order to output the phenotypes directly from raw images.
- Project 3.2 covers various other topics such as plant staging, which is using the quantity of plant information in a picture to detect specific growth stages.
Some of the new tools developed within 3.2 include a stationary imaging system for taking time lapse pictures of a field (COASt), and the "GrowPro," another camera on a stick used for imaging by walking through a field. These can be seen below, as well as an illustration of field imaging and remote sensing, which shows some of the data collected from these imaging methods.
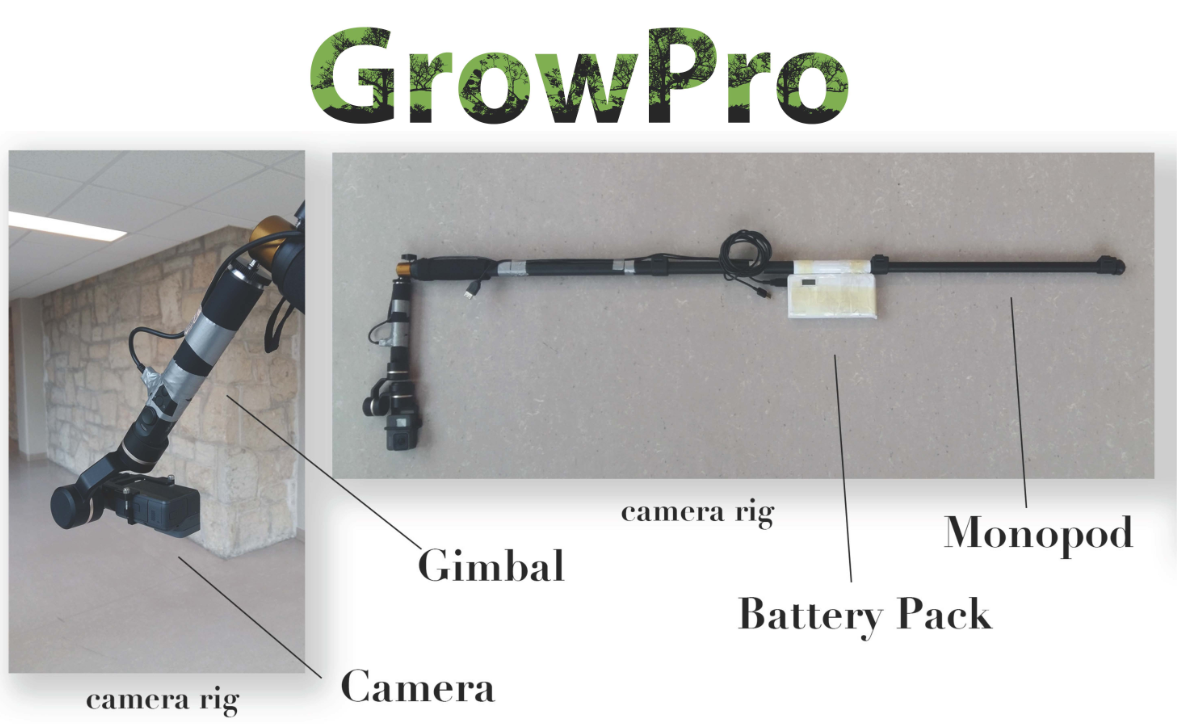
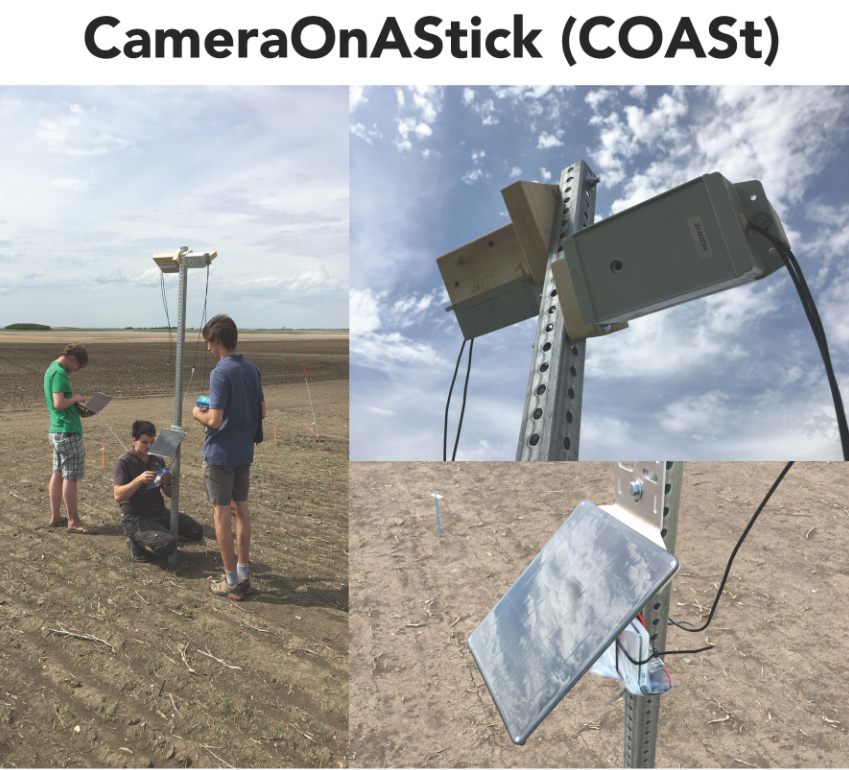
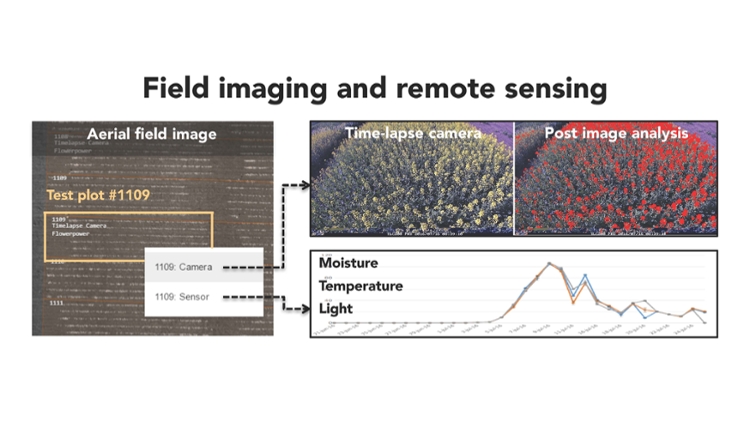
Practical Applications
- Commercial breeders are faced with the same challenges as academic breeders, except on a larger scale. By collaborating with researchers, they will have access to new and productive advances in phenotyping.
- Farmers may benefit from the use of these technologies and data collection in the future. An example of this would be by utilizing drone imagery to analyze growth stages of crops, or to assist in making more informed management decisions (like when to use fertilizer or pesticides) with less effort.
- Computer recognition has the potential to increase the speed, reliability, and precision of trait identification, currently done manually in the field. Plant breeders can collect data more quickly and frequently, so they truly have the information needed to select the best traits. This automation will provide new opportunities in plant science and breeding to directly compare large quantities of individual crop plants with known genetic differences.
Collaborations
Project 3.2 works in collaboration with various other P2IRC projects:
- Project 1.1: Phenotype to Genotype: Moving from Model Systems to Crops is looking at phenotyping in a controlled indoor environment and provides plant information for Project 3.2 to process.
- Project 1.2: Leveraging Field Phenomics for Advancing Key Rotational Crops uses mobile vehicle platforms for imaging crops, then shares these images with Project 3.2 in order to analyze the data.
- Project 2.3: High-Throughput Data Acquisition for 3D Structural Imaging and Characterization of Plant Phenotypes images roots and microbial communities, which Project 3.2 then processes.
Research Team
Loading...
Project Co-Lead:
Mark Eramian
Research Associate:
William van der Kamp
Post-Doctoral Fellows:
Tuhin Paul
PhD Students:
Masi Aslahi
Jordan Ubbens
MSc Students:
Rahat Yasir
Hao Song
Imran Ahmed
Anupama Das
Ilya Ovsyannikov
Javier Garcia
Aich Shubhra
Travis Gray
Undergraduate Students:
Keenan Johnstone
Christopher Chamberlain
Kyle Seidenthal
Connor Lavoy
Colby Lemieux
Blanche Leyeza
Sajith Rajapaksa
Danny Huang
Publications
- Ubbens JR and Stavness I. (2017). Deep Plant Phenomics: A deep learning platform for complex plant phenotyping tasks. Frontiers in Plant Science. 8: 1-10. https://doi.org/10.3389/fpls.2017.01190
- Aich S and Stavness I. (2017). Leaf counting with deep convolutional and deconvolutional networks. IEEE International Conference on Computer Vision, Computer Vision Problems in Plant Phenotyping workshop. pp. 1-10, Oct. 2017, Venice, Italy https://arxiv.org/pdf/1708.07570